
Newsletter
Special Interest Group of the American Association of Bioanalysts (AAB)
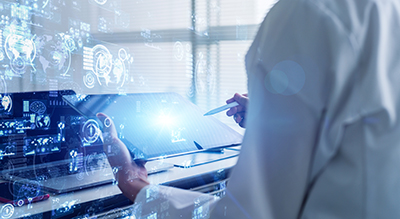
Embryology 3.0: ARTs of the Future will be Guided by Artificial Intelligence Systems
by Carol Lynn Curchoe Burton, Ph.D, TS(ABB)
CCRM Network, Newport Beach, and ART Compass
Millions of babies have been born through Assisted Reproductive Technologies (ART), however, only 30% of IVF cycles succeed in a clinical pregnancy. Aside from increasing the success rate, there are other worthwhile goals for continued improvement across the industry; to simply get patients pregnant faster, reduce treatment dropout, or to reduce embryo wastage. Innovations in Artificial Intelligence (AI) will drive ART that is more reproducible, standardized, efficient, and less costly. However, clinical ART labs are almost totally unprepared to implement AI systems.
There are significant challenges to implementing any AI system in a meaningful way into a clinical IVF laboratory, yet it is almost certain that AI is the future of reproductive medicine. Wherever electronic health records (generally), and AI systems specifically have been proposed, there are unresolved controversies about privacy, effects on doctor/patient relationships and trust, whether individuals can opt out, who controls or can have access to data and when consent is required, how to protect data from unauthorized use or accidental loss, and use of "de-identified" data for ethically approved research.
Implementation of an AI at the actual point of care in a routine, easy, and automated fashion is also currently impossible for the majority of ART clinics that still use paper charts, and don't capture and store even a single digital image of their patient's embryos or gametes, much less the videos that are needed for true big data. Additionally, data management solutions to augment patient demographics, clinical and lab key performance indicators (KPI) and other relevant data streams (ultrasound images and PGT results, competency assessments, endocrinology, microscope and incubator QC, room/environmental factors) into a single dashboard, are lacking.
The development of AI systems that are widely applicable across ART clinics and protocols, AND geographies and populations have been challenged by the quality, diversity and volume of available data. AI systems need big data to achieve robustness. Accessing so-called "BIG" data for ART should be a trivial problem, as more than 2.7 million assisted reproduction cycles are performed each year.
Research laboratories worldwide (see www.repro-ai.org for updated publications list) have developed AI systems to enhance our diagnostic and prognostic capabilities, minimize variability and error, and maximize precision and efficiency of IVF treatment, but they have not yet achieved robustness for safe clinical implementation, and no prospective validation trials have been published yet. A lack of early stage capital infusions to bolster innovation in ART is delaying getting these innovations out of academic labs and early stage start ups, and into routine patient care.
Another challenge we face in AI development is the quality of the training data and how the machine learns from it. The training data- perhaps embryo, oocyte, or sperm images- still have to be evaluated and graded by human embryologists, and the populations these training data come from may be biased (by income, race, infertility diagnosis, etc.). How well the AI learns depends on the quality and size of the dataset.
It is likely that the majority of AI training data are generated mainly from IVF cycles in a very specific patient population- white, wealthy, etc. There is a marked, and unacceptable, difference in the outcomes after fertility treatment between minority populations. The assisted reproductive technology failure (ART) rate, defined as no live birth after treatment, is 51.9% (white), 61.8% (Asian), 62.2% (Black) and 55.9% (Hispanic) and ART stillbirths account for 16.3% (white), 18.4 (Asian), 25.0% (Black) and 17.8 (Hispanic).
These are problems that AI is uniquely suited to solve. Artificial intelligence in "precision reproductive medicine" has the potential to resolve the genetic uniqueness of individuals and the molecular mechanisms of their infertility, to match each individual to the best treatment.
Conventional IVF (despite scientific advances like genetic carrier screening, embryo biopsy with pre-implantation genetic diagnosis, ICSI and "freeze all" cycles) is woefully inefficient and wastes millions of gametes and embryos worldwide. Convolutional neural networks have been shown to choose genetically "normal" blastocysts from single static images or video, better than fully trained embryologists with dozens of years of experience. Advances like these, when applied clinically, have the potential to address this problem.
In the U.S. there is a vast underserved infertility market that includes those seeking genetic disease prevention, LGBTQ, proactive fertility management (oocyte and sperm freezing), and those without access to fertility care (ie. most people, as only 16 states require infertility insurance; although private employers are increasingly offering these benefits). We know beyond a shadow of a doubt this is true, by comparison with other developed countries. There are 7 million women with infertility in the U.S., and only 1% of those successfully deliver an in vitro fertilization baby per year. Australia, Japan, and many parts of Europe have rates that are three times higher than the U.S. IVF is an incredibly manual labor-intensive process.
There is a dearth of well-trained embryologists to perform these labor-intensive tasks and staff training is often a "bottleneck" for IVF labs. AI systems have the potential to revolutionize the market by assisting with the manual, time and labor, rote, routine, intensive and subjective, embryology tasks–and increase the number of patients (scale) we can serve safely. That is if we if we can put aside the unwarranted fear that computers are going to take over our jobs, and give artificial intelligence the chance to help us.
Free Webinar ⇒CRB News Articles
12/10/2020
Vol 9, No. 3
12/10/2020
From the President and Committee Chairs
12/10/2020
IVF in the Time of Corona
12/10/2020
Comprehensive Training for your IVF Lab
12/10/2020
Embryology 3.0: ARTs of the Future will be Guided by Artificial Intelligence Systems
12/10/2020
AAB Free Recorded Webinars
12/10/2020
25th Annual CRB Symposium
12/10/2020
CRB Free Recorded Webinars
CRB Standing Rules - Log in to view